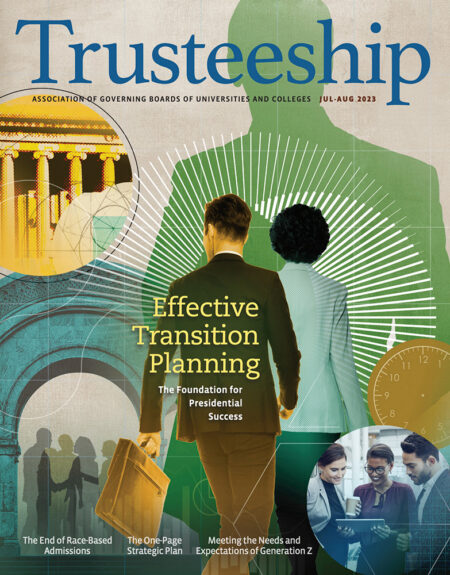
For colleges and universities to realize the potential value of the “Data Science Internal Internship” concept, the Brandeis University experience so far shows the necessity of four key ingredients:
- Champions at the top: Explicit support by the Executive Vice President of Administration and Finance and also by the Provost, encouraging administrators to consider participating in the DSI Internship;
- Stamp of approval by relevant faculty of student finalists: Relevant faculty dedicated to vetting top performing students for technical mastery, problem solving creativity, and personal trustworthiness and integrity;
- Infrastructure of on-going support and quality control: weekly meetings with the DSI Intern cohort, regular check-ins with participating administrators, and open communications with data governance policy leaders;
- Local control: Intern selection from pool of finalists, as well as project scope is defined locally, within the divisions overseen by participating administrators; this is a necessary form of support for those administrators willing to devote mentoring time to Interns, and to open their operations to new technologies with which they might not be familiar.
SUMMARY
Undergraduates working as data science interns in university administration can help speed-up and broaden the effective implementation of new technologies supporting datainformed decision-making, a critical need for developing and using metrics to drive strategic success.
Higher education has a problem when it comes to data science. It generates massive amounts of data. It also faces tremendous need to transform that data into strategic support for decision-making across the university. But in general, higher education continues to lag behind other sectors in putting its data to work. As one team of analysts recently put it, “universities are data rich and data poor—and sometimes intentionally data blind.”1
Fortunately, this gap between opportunity and need is increasingly getting the attention it deserves, with researchers zeroing in on the structural sources of the problem, professional associations delivering practical guidance on how to overcome them, and practitioners highlighting cutting-edge examples of the great value that results when university operations integrate data scientific tools successfully.2 We have good news to add to this positive momentum: Undergraduates working as data science interns in university administration can help speed-up and broaden the effective implementation of new technologies supporting data-informed decision-making.
Two and a half years ago, we launched a pilot “internal internship” program at Brandeis University. With the support of the university’s leadership team, including the executive vice president for finance and administration and also the provost, our aim was to build a bridge linking the supply and demand of data scientific talent within the university, connecting high-performing undergraduates with willing administrators. The concept for this pilot program emerged from our research on the higher education workforce, where we focused specifically on the demand for data scientific skills.3 Our findings identified research universities as one of the fastest growing centers of data scientific talent over the decade prior to the COVID-19 pandemic. This was true both for faculty hires—where we saw that data science, machine learning, and artificial intelligence were leading the way in computer science job openings—and also for staff hires—where research universities showed faster growth in demand for data scientific skills than did the U.S. economy as a whole. In other words, by considering overall the human capital represented by staff and faculty (and their students), we could see that research universities are uniquely positioned to address their own data scientific needs.
To connect the dots, and bring students’ data scientific talent to a variety of data scientific demands within university operations, we needed to create an infrastructure of on-going support and quality control. We started by asking each willing administrator to write a job description for their projects, to help clarify the scope of the problem, articulate the value to the office of the hoped-for outcome, and specify the nature and location of the relevant data. We relied on computer science faculty to create a vetting process for defining the universe of eligible students, which would require evidence of excellence in academic performance, curricular relevance, and trustworthiness. We understood that administrators themselves would need to be the ones making the final selections: Administrators needed the chance to evaluate students for proper personality fit for their offices while relying on faculty to confirm technical expertise and conceptual creativity.
On the student side, we required a group meeting on a weekly basis, thereby creating a cohort among those in the Data Science Internal (DSI) Internship. Group discussions help each DSI Intern stay on track with project objectives through the weekly status reports that characterize each meeting. Specifically, these discussions include guidance on technical questions and innovation opportunities, and help students navigate working relationships with supervisors and office peers. We require careful attention to university protocols on data security. We examine the potential relevance and risks of technologies that Interns might consider introducing. We call upon Interns to share not only insights from their work but also ways of ensuring that their work has impact. For example, one student described the value of translating important results of analyses they achieved through Python back into Excel because Excel is the analytics tool used most broadly in the affected office. We review licensing issues that represent key constraints or benefits in using one set of data scientific tools over another. We continually ask each Intern to prepare appropriate documentation of their work, creating on-boarding materials for successor DSI interns, full-time staff, or consultants to pick up where current interns leave off.
The more experience we acquire with the data science internal internship concept, the more we learn about the features essential to its success. A passion for learning among the DSI intern cohort, where students take responsibility for teaching themselves the basics of new skills they might need to solve a problem, or immerse themselves in the details of a particular administrative field in order to better understand the office objectives of their mentor, always seems to be a key driver of productivity. Without it, we have seen less effective outcomes from the relationship between DSI interns and their administrative mentors. Similarly, a commitment from administrators to regular engagement, where problems and their solutions are defined, implemented, evaluated, and amended quickly, seems to make all the difference in creating useful results. This rapid-fire, iterative mode of working together means that administrative mentors—the ones responsible for defining required outcomes—are close to the technical challenges of new applications while the DSI interns—responsible for implementing data scientific solutions—are close to the demands of operational effectiveness. The results from this “scrum” mode of innovation are practical solutions that help educate students and administrators at the same time, keeping them aligned on the possibilities of worthwhile impact.4
Our initial results have been promising. Over the past 30 months, our DSI interns have jump-started the visualization, analysis, and accessibility of reliable data in several divisions: In human resources, they helped launch a dashboard of HR metrics such as turnover, time-to-fill, and recruitment pool diversity. In academic administration, they created interactive visualizations capable of showing multi-year trends in course enrollments, faculty teaching workloads, and student declarations of majors and minors. In research administration, they are making it easier to distribute interactive information to senior leaders about the university’s track record in grant applications and research funding, broken down by faculty member and department as well as by funding source. They are also designing predictive tools to help evaluate the likelihood of a proposal’s succcess.
In addition to creating working dashboards from multiple sources of university data, DSI Interns have built prototypes of predictive models for charitable giving from alumni. They have built the infrastructure of complex statistical analysis necessary to explore potential demographic bias in course evaluations. They have designed tools capable of analyzing legacy data from campus operations in order to identify trends in building alarms and energy usage and prioritize the response of facilities workers. They have created interactive maps of the university’s steam pipe network, thereby minimizing uncertainty for administrative leaders in facilities planning and maintenance. They have also helped broaden Information Technology Services’s capacity to support the implementation of Brandeis’s cloud-based enterprise management system by learning to generate data analytic reports, experimenting with some of the system’s newest functions, and helping to pinpoint the source of some of the system’s key shortcomings.
From the point of view of undergraduates learning data science at Brandeis, and the faculty who teach them, the DSI Internship concept carries distinctive educational value (as most experiential learning opportunities do): Students are given the opportunity to join a team of professionals, and to apply in a mission-critical environment, those concepts, tools, and techniques that they have been learning in class. Add to that the unusual benefit of learning the nuts and bolts about the workings of their school, realizing they are making an important contribution to its success, and it is no surprise that interns, their faculty, and employers who interview DSI interns for jobs after graduation are all well aware of the unique worth of the DSI Internship learning experience.
Top performing students can help drive innovation and increase operational effectiveness when it comes to data scientific applications in higher education. The Data Science Internal (DSI) Internship project at Brandeis University explores this possibility by linking relevant faculty, top performing students in data science, and willing administrators across the university open to allowing student Interns to bring data scientific solutions into existing work-flows. Many administrators of operational divisions have decades of experience with student workers. But the DSI Internship is different in the depth of skill it expects from the Interns, and in the dedicated mentoring it expects from participating administrators—specifically on matters of domain knowledge and of the organizational dynamics associated with achieving office objectives. Most distinctly, the Internal Internship concept assumes that technical knowledge transfer will occur from the interns to their respective offices in ways that support operational innovation and effectiveness. The DSI Internship is aligned with multiple components of the university’s mission: excellent educational opportunities for students, improved post-graduate job options for students as a result of experiential learning preparedness, and more data-informed decision-making in university operations that leads to better outcomes.
Less obvious has been the embracing response of our administrative partners. It would make sense for administrators responsible for a division to be reluctant to trust an undergraduate with their division’s data, or with data reporting responsibilities, even if that student were already vetted by relevant faculty as especially capable and accomplished. It would also make sense for administrators to feel a bit uncomfortable about asking an undergraduate to introduce new technologies for analysis when the administrators themselves are not familiar with those technologies. Fortunately, we have found the opposite response.
Our administrative partners are proud of the technological sophistication that allows their DSI interns to pull insight out of data that has not yet been subject to data scientific analysis. They value the increased relevance that senior leaders find in their data, thanks to analytic and presentation techniques introduced by their Interns. They welcome the opportunity to mentor and teach their students about the missions of their respective offices, creating customized decision-support tools together along the way. Our administrative partners have become the biggest advocates for encouraging other administrators at the university to place a DSI intern on their teams. More importantly, their advocacy has encouraged the university’s Data Governance Program (which is responsible for campus-wide policies governing access, usage, and security of university data) to develop security protocols enabling DSI interns to see each other’s data. This now allows them to safely bypass organizational silos in order to share and learn from each other’s experiences in data scientific applications.
How would we know if we’re being successful? We have some anecdotal results. We’ve seen administrative mentors hire their graduating DSI interns into staff positions, to continue the data scientific work they began as students. We’ve seen our administrative partners use visualizations and analytics generated by their DSI interns, that had not been available before, to illuminate strategic insights for their own supervisors, including for the president and the board of trustees. We’ve seen administrators work with existing vendors of data analytics to begin exploring ways of amending their products in support of systems built by DSI interns. We know that our first foray into this project, our exploratory phase, now justifies a new phase, where we will focus on creating tools and metrics for evaluation, accompanied by illustrative examples to help formalize what we’ve learned.
Admittedly, the wins we’re experiencing through this internship program are mostly quick wins, only possible because the higher education sector is behind the curve. Our program is still a pilot project, an exploration of a concept, just touching the tip of the data science applications iceberg. Yet, we have noticed the emergence of a new kind of trust among administrators, students, and faculty: Accomplished students and motivated administrators can indeed come together around a shared commitment to teaching and learning from each other to help bring university data to life. Overall, we believe that the DSI Internship at Brandeis, together with others that might replicate it at other institutions, have the potential to inspire an industry-wide mindset of optimism. We see an openness to the possibility of success when it comes to the digitization of higher education
Jessica Liebowitz, Ph.D., is a research scholar in computer science at Brandeis University where she helped launch the Data Science Internal (DSI)
Internship.
Tim Hickey, Ph.D., is a professor of computer science at Brandeis University, where he focuses on educational technology, brain-computer interfaces, and game-based learning.
End Notes
1 Christine Borgman and Amy Brand, “Data Blind: Universities Lag in Capturing and Exploiting Data,” Science 378, no. 6626 (December 23, 2022).
2 Center for Education Policy Research, “Strategic Data Use in Higher Education: Improving Postsecondary Success with Data,” (Cambridge: Harvard University, June 2020); “Joint Statement on Analytics,” Association for Institutional Research (AIR), EDUCAUSE, and National Association of College and University Business Officers (NACUBO) joint press release (August 28, 2019); follow-up Actionable Guide: “With $500,000 Grant, Higher Ed Associations Will Help Colleges and Universities Accelerate Analytics Use,” AIR, EDUCAUSE, and NACUBO joint press release (November 10, 2022); Karen Webber and Henry Zheng, eds., Big Data on Campus: Data Analytics and Decision Making in Higher Education (Baltimore: Johns Hopkins University Press, 2020).
3 Jessica Liebowitz, Tim Hickey, and Matthew Ekins, “Exploring the Impact of Computer Science on the Future of Higher Ed,” Data Science Initiative Series, Fall 2021, Brandeis University.
4 Ever since publication of the “Agile Manifesto” in 2001, www.agilemanifesto.org, software development methodologies focusing on flexibility, self-organizing work teams, and closer engagement with the client have flourished across industries. One such framework, “scrum,” specifically aims to better serve customer needs through continuous improvement and rapid iteration among team collaborators; see Sean Peek, “What Is Agile Scrum Methodology?” Business News Daily (February 21, 2023).
RELATED RESOURCES
Trusteeship Magazine Article
The College of the Future, Part 1
Reports and Statements
AGB Board of Directors’ Statement on Innovation in Higher Education