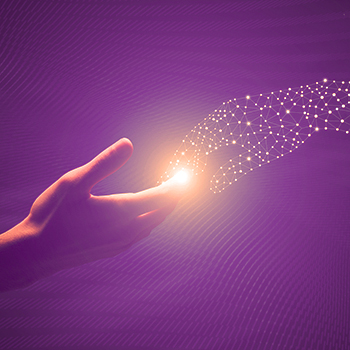
Opinions expressed in AGB blogs are those of the authors and not necessarily those of the institutions that employ them or of AGB.
The recent, rapid advances in artificial intelligence (AI) have created hype that can pose quandaries for advancement leaders and board members who are looking to explore the potential opportunities for their higher education institutions. This blog aims to provide some clarification around different types of AI and how different applications can provide value to higher education advancement shops.
First Things First: What Is AI?
Confusion around this question arises because AI is somewhat of an umbrella term. Asking someone, “What is AI?” is about as broad a question as asking, “What is art?” At a high level, AI is often defined as the simulation of human intelligence using machine models that are trained to recognize patterns in data.
The term AI doesn’t refer to any particular discipline, application, or value proposition. It can refer to robotics, natural language processing, computer vision, or fuzzy logic, all of which are different types of AI that are used in many applications most of us are familiar with today.
AI is your Google Home, your Amazon Alexa, your face ID on your iPhone, or your Roomba vacuum cleaner. So, despite its recent popularity, AI has been around for a while, and for most of us, it is integrated into our daily lives in one way or another.
Generative AI
Generative AI deserves a special mention because it is responsible for causing all the buzz of late. It is important to note that generative AI serves the specific purpose of generating content. ChatGPT and DALL-E are two of the most well-known applications of generative AI, and they use natural language processing to generate new text and images in response to a prompt. But most practical uses for AI actually innovate in fairly straightforward ways.
Machine Learning
Machine learning (ML) is a branch of artificial intelligence, often defined as a field of study that allows computers to learn without being explicitly programmed. At some level, most AI applications will utilize ML, and it has been used in enterprise applications to great success in many industries.
For example, Whistler Blackcomb, a ski resort in Canada, uses ML to predict with high accuracy what the weather will be and how busy the hotel will be in order to help staff its operations efficiently. Using AI/ML, Whistler Blackcomb is able to vastly improve its decision-making compared with the traditional methods it previously used.
At the same time, many retail companies have used ML in customer loyalty programs to provide insights on things such as what coupon to send next to get a customer back into their stores.
For advancement organizations, AI/ML can highlight opportunities to improve decision-making and create operational efficiencies by lifting a manual burden. AI/ML can help decision-making by providing predictions such as who will make a major gift in a certain time frame, who is likely to increase their giving to a certain threshold, or who is likely to lapse.
So How Does It Work?
In order to build machine models that work, you need a lot of data, and the datasets that are used have to be specialized to the question that is being asked. So organizations need to start capturing these data in an automated way in order to build their datasets for now and the future.
Machine models are trained using millions or even billions of examples. They are encouraged when they are correct and discouraged when they are wrong, until they become exponentially smarter the more they are used. Humans provide feedback about the outcomes of the model, that feedback is incorporated into the training process, the model is fine-tuned, and that process is repeated.
Is Automation AI?
Automation and AI are not the same thing, although the technology is complementary. Automation is a form of traditional software programming that follows pre-programmed rules as opposed to ML, which is not explicitly programmed. However, automation is a core part of ML, making ML processes faster and more efficient.
New Thinking
The world is changing, and so are the expectations of the next generation’s students around their relationships with their schools and their engagement with brands. Intelligent AI systems are flexible enough to adapt to these changes, and they have benefits from an engagement, cost, and regulatory perspective.
Data Collection
- Data are non-renewable resources, and data that are captured in the context of time are data that an organization cannot get back in the future if it doesn’t start capturing them now.
- Machine learning techniques help streamline data collection and convert data into a standard format.
- Demographic and donation data don’t cut it anymore, but current systems don’t capture behavioral data and timing data.
- Capturing behavior, interests, and preferences allows organizations to engage alumni with communication that is relevant to them.
Data Ecosystem: Connecting Siloed Data Systems
- Intelligent systems allow you to automate your activities based on alumni and stakeholder preferences.
- Often, customer relationship management (CRM) functions as the center of foundation operations and the source of truth. It doesn’t (and can’t) consume all data sources, leading to silos and lost/desynced data.
- An intelligent data layer can act as a centralized hub for communications between an organization’s systems, of which CRM will be one spoke.
- This layer can use the legal name of a person on a tax receipt but their nickname in casual correspondence. Or it can join data together to see the critical interactions with someone across systems but break apart the data when an organization needs to show certain data points to certain staff members and not others.
- This approach increases flexibility and decreases the cost of achieving the same flexibility through traditional approaches.
Personalization
- The next generation of donors expect the brands they support to know what they care about and consider their preferences.
- Individuals have endless options for how they spend their time, and personalizing the alumni and stakeholder experience is key to the future.
- Organizations can start to personalize their engagement with alumni and stakeholders, and build better segmentation of whom they reach out to and what message to communicate to them.
- AI can remember individual preferences and personalize the experience for each donor.
- Organizations should automate activities based on constituent preferences rather than internal constraints.
Future-Proofed Data Governance: Flexible to Change with Legislation
- Flexible technology systems can allow organizations to match internal controls with regulatory obligations. A good example of this is the recent change in privacy legislation in many states that will require organizations to change how they are handling constituent data.
- Constituent preferences from granular to broad can be reconciled in a central location across an organization’s multiple systems.
- Technology systems can manage access and permission controls for individuals and different business units and teams in an organization.
- Soundly architected infrastructure can provide redundancy benefits and cost optimization while simultaneously providing the ability to comply with data residency requirements.
Attracting and Retaining the Next Generation of Talent
- Machines can do the work that machines do best, and humans can do higher value work such as building relationships. This means less manual drudgery for staff.
- Human discipline is important, but automation can greatly assist in managing millions of data points.
Keeping Humanity at the Heart of AI
Other important considerations in the use of AI are the introduction of bias and the importance of proper data compliance. Many frameworks are evolving to help humanity deal with bias and data compliance, but these frameworks must be tuned to meet the needs of each organization. One of the complexities of compliance and bias is that there are nuances. In order to give these topics proper attention, we look forward to more in-depth content review in the future.
It is clear that the AI gap in higher education is a transformational opportunity for the sector, and for the students and stakeholders it serves. Humankind has never had a chance to go so far or so fast as it does with AI. Just as AI needs a lot of data, this conversation needs a lot of voices. We hope you’ll join it.
Rachel Crosbie is vice president of operations and strategy at Fundmetric.
With Thanks to AGB Mission Sponsor: Fundmetric
RELATED RESOURCES
Trusteeship Magazine Article
Foundations of Consequence: Board Leadership, Philanthropy, and Supporting Higher Education as a National Asset